Safeguarding Digital Frontiers: AI in Cybersecurity for Advanced Threat Defense
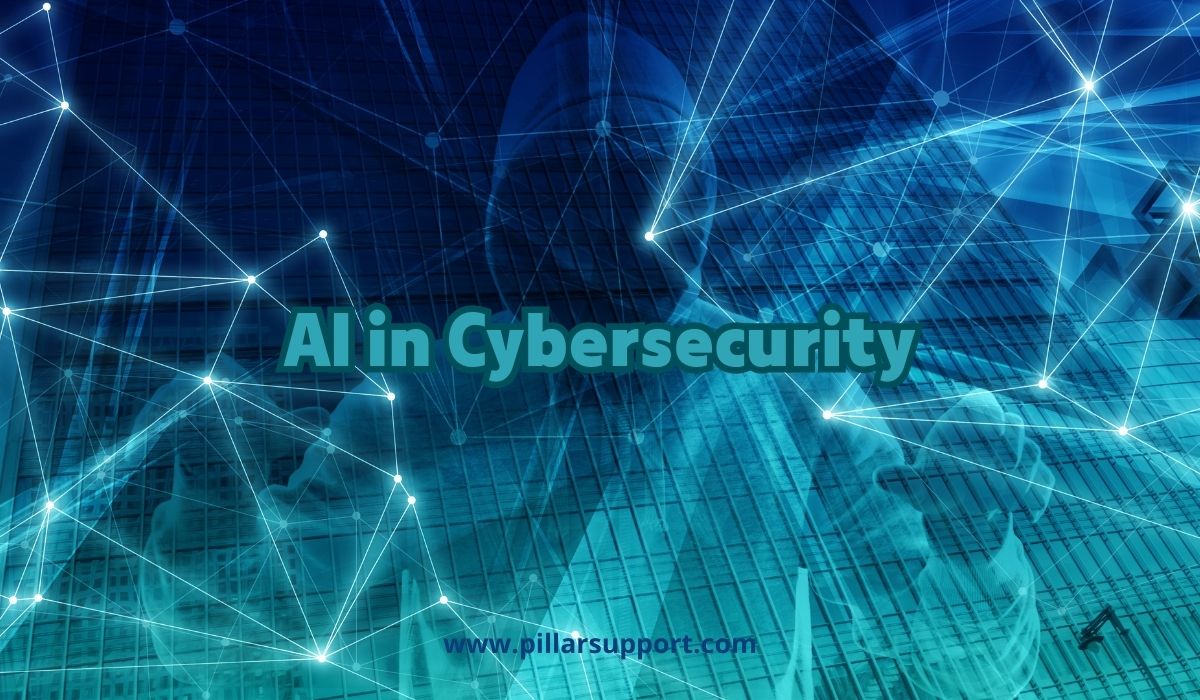
In today’s fast-paced digital landscape, the importance of AI in cyber security for modern businesses cannot be overstated. With the increasing frequency and sophistication of cyber threats, businesses are facing a pressing need to implement advanced defense mechanisms that can effectively safeguard their sensitive data and critical systems.
As cybercriminals continually adapt their tactics, AI has emerged as a game-changing tool that empowers organizations to stay one step ahead in the ongoing battle against cyber threats.
Let’s delve into the world of AI-powered cybersecurity and understand how it is reshaping the way businesses protect their digital assets.
Table of Contents
Understanding AI in Cyber security
In the realm of cybersecurity, AI-driven solutions are redefining the way businesses protect their digital assets. AI, or Artificial Intelligence, encompasses a range of technologies that enable systems to perform tasks that typically require human intelligence, such as problem-solving, pattern recognition, and decision-making. In the context of cybersecurity, AI is harnessed to bolster defense mechanisms against an ever-evolving landscape of cyber threats.
At the heart of AI-driven cybersecurity are machine learning and deep learning techniques. These technologies empower computer systems to learn from data and improve their performance over time without being explicitly programmed. Machine learning algorithms can detect patterns and anomalies within vast datasets, enabling them to identify potential threats and vulnerabilities that might go unnoticed by traditional security measures. Deep learning, a subset of machine learning, uses neural networks to simulate the human brain’s complex structure, making it highly adept at recognizing intricate patterns and nuances in data.
By leveraging these AI technologies, businesses can fortify their cybersecurity defenses, detect emerging threats in real-time, and proactively respond to potential breaches. This marks a pivotal shift in the cybersecurity landscape, as AI augments the capabilities of traditional security measures and helps organizations stay ahead of cyber adversaries.
AI-powered Threat Detection
One of the most significant contributions of AI to cybersecurity is its ability to provide real-time anomaly detection and behavior analysis. Traditional rule-based security systems often struggle to keep up with the dynamic nature of cyber threats. AI-powered systems, on the other hand, excel at identifying deviations from normal patterns of behavior, making them incredibly effective at detecting previously unknown threats.
AI-driven threat detection systems continuously analyze vast amounts of data from network activities, user behaviors, and system logs. By establishing a baseline of normal behavior, these systems can swiftly identify any deviations that might indicate a potential breach or attack. For instance, if a user suddenly accesses an unusual set of resources or a device starts sending a significant amount of data to an unknown location, the AI system can flag these activities as suspicious.
Furthermore, AI plays a crucial role in threat intelligence. By analyzing massive volumes of data from various sources, including the dark web, AI can identify emerging threats, zero-day vulnerabilities, and potential attack vectors. This proactive identification of risks allows organizations to take preventive measures and strengthen their defenses before any attack occurs.
The synergy of AI-powered threat detection, real-time anomaly analysis, and threat intelligence equips businesses with the capability to identify and respond to threats rapidly, significantly reducing the risk of data breaches and unauthorized access to sensitive information.
AI in Endpoint Security
Endpoint security, which focuses on protecting individual devices or endpoints within a network, has greatly benefited from AI-powered solutions. These solutions provide advanced protection against a wide range of cyber threats, including malware, ransomware, and phishing attacks.
AI-powered Endpoint Protection and Detection Response
Traditional signature-based antivirus solutions are limited in their ability to detect new and evolving malware. AI-driven endpoint security solutions, however, use machine learning algorithms to continuously analyze and learn from new threats. This enables them to identify and block malicious activities in real time, even if the malware is previously unseen.
These solutions go beyond just recognizing known patterns of attack. They can identify suspicious behaviors that might indicate a threat, such as unusual file access, unauthorized processes, or attempts to tamper with system files. By using AI to establish baselines of normal behavior, these solutions can detect deviations and respond swiftly.
AI-driven Malware and Ransomware Detection and Prevention
Malware and ransomware attacks are constantly evolving, making them particularly challenging to detect and prevent. AI-powered solutions excel at identifying patterns and behaviors associated with these threats.
For instance, AI algorithms can analyze the behavior of files and applications to determine if they exhibit characteristics of malware. They can also monitor network traffic for signs of data exfiltration, a common tactic of ransomware attacks.
In the case of ransomware, which encrypts a victim’s data and demands payment for its release, AI can detect the encryption process as well as the attempt to communicate with command and control servers. This allows for quick response to isolate the affected device and prevent further damage.
AI in endpoint security not only enhances protection but also reduces false positives, enabling security teams to focus their efforts on genuine threats. By leveraging AI-driven solutions, businesses can fortify their devices against both known and emerging cyber threats, safeguarding sensitive data and maintaining operational continuity.
AI and Network Security
Network security, a crucial aspect of cybersecurity, has greatly benefited from the integration of AI technologies. AI-powered solutions enhance the ability to detect and respond to complex cyber threats in real time, ensuring the integrity and confidentiality of sensitive data.
AI-enabled Network Traffic Analysis and Intrusion Detection
Traditional network security solutions often struggle to keep up with the scale and complexity of modern cyber threats. AI-driven network security solutions utilize machine learning algorithms to analyze network traffic patterns, identify anomalies, and detect potential intrusions.
By learning from historical data and identifying deviations from established patterns, these solutions can quickly recognize suspicious activities, such as unauthorized access attempts or data exfiltration. AI algorithms can also distinguish between normal and abnormal user behaviors, reducing false positives and enhancing the accuracy of threat detection.
AI-driven Threat Hunting and Network Anomaly Detection
Traditional security methods rely on predefined rules and signatures, which can be bypassed by sophisticated attackers. AI-powered network security takes a proactive approach by hunting for signs of advanced threats that might evade traditional defenses.
AI-driven threat hunting involves analyzing vast amounts of network data to uncover hidden threats and malicious activities. This can include identifying unusual patterns in traffic, unauthorized lateral movement within the network, and attempts to exploit vulnerabilities.
AI-driven network security solutions continuously adapt and learn from new threats, enabling them to identify and respond to previously unknown attack vectors. This is particularly crucial in defending against zero-day exploits and polymorphic malware.
By leveraging AI in network security, organizations can achieve more effective threat detection, rapid incident response, and enhanced protection against a wide range of cyber threats. The combination of AI’s pattern recognition capabilities and real-time analysis provides a powerful defense against attacks that aim to compromise network integrity and compromise critical systems.
AI in Email Security
Email security remains a significant concern for businesses and individuals, with cybercriminals using various tactics to launch phishing attacks, spread malware, and gain unauthorized access to sensitive information. AI has played a crucial role in enhancing email security by providing advanced threat detection and response capabilities.
AI-powered Spam Filtering and Phishing Detection
Traditional email security solutions often rely on rule-based approaches to filter out spam and detect phishing emails. However, cybercriminals have become increasingly sophisticated in crafting deceptive emails that can evade these rules. AI-powered email security solutions utilize machine learning algorithms to analyze email content, sender behavior, and other contextual information to identify potential phishing attempts.
By learning from large datasets of legitimate and malicious emails, AI algorithms can recognize patterns and indicators of phishing attacks that might not be easily detected by rule-based methods. These algorithms can adapt to new and evolving phishing techniques, making them highly effective in mitigating the risks associated with phishing.
AI-driven Email Threat Analysis and Malware Detection
Emails are a common vector for malware distribution, including ransomware, trojans, and viruses. AI-driven email security solutions analyze attachments, links, and email content to identify potentially malicious elements. These solutions can detect malware by recognizing suspicious patterns, file signatures, and behavior associated with known malware strains.
AI can also analyze the email sender’s behavior and history to determine the likelihood of an email being malicious. For instance, if an email claims to be from a legitimate sender but exhibits behavior inconsistent with their past communications, AI algorithms can raise a warning.
Implementing AI in email security significantly reduces the risk of falling victim to phishing attacks, malware distribution, and other email-based threats. By leveraging AI’s ability to analyze patterns and behaviors, organizations can better protect their email systems and sensitive information from cyber threats.
AI and Cloud Security
Cloud security is a critical concern as more businesses migrate their operations and data to cloud environments. AI has emerged as a powerful tool in enhancing cloud security by providing advanced monitoring, incident response, and data protection capabilities.
AI-based Cloud Security Monitoring and Incident Response
Traditional security monitoring in cloud environments often involves rule-based systems that generate alerts based on predefined patterns. However, these systems may struggle to detect complex and evolving threats. AI-powered cloud security solutions utilize machine learning algorithms to analyze massive amounts of data generated by cloud applications and services.
These algorithms can identify unusual patterns, behaviors, and anomalies that may indicate a potential security breach. By continuously learning from data, AI systems can adapt to new attack vectors and evolving threats, enabling faster and more accurate detection of security incidents.
Additionally, AI-driven incident response can help automate the process of analyzing and mitigating security breaches. AI systems can assess the severity of an incident, recommend appropriate actions, and even carry out automated responses to contain the threat.
AI-driven Data Protection and Encryption in the Cloud
Data protection is a paramount concern when using cloud services. AI can enhance data security by providing robust encryption and access control mechanisms. AI algorithms can analyze user behavior and access patterns to detect unauthorized access attempts and potential data breaches.
Moreover, AI-driven encryption techniques can dynamically adapt to different threat scenarios. For example, if a user’s access behavior deviates from the norm or if unusual activities are detected, the AI system can trigger additional layers of encryption to protect sensitive data.
AI’s ability to analyze large datasets and detect subtle patterns makes it a valuable asset in securing cloud environments. By leveraging AI-driven monitoring, incident response, data protection, and encryption, organizations can better safeguard their cloud-based assets and sensitive information from cyber threats.
AI for Cyber Threat Intelligence
Cyber threat intelligence involves collecting, analyzing, and interpreting data to identify potential cyber threats and vulnerabilities. AI has significantly enhanced the effectiveness of cyber threat intelligence by enabling faster and more accurate data analysis, automated threat detection, and proactive incident response.
AI-powered Threat Intelligence Platforms and Data Analysis
Traditional methods of threat intelligence often involve manual data collection and analysis, which can be time-consuming and limited in scope. AI-driven threat intelligence platforms can automate the process of collecting and analyzing vast amounts of data from various sources, including open and closed web forums, dark web marketplaces, and social media.
Machine learning algorithms can identify patterns, trends, and indicators of compromise that human analysts might overlook. This enables organizations to gain a comprehensive view of potential threats, vulnerabilities, and attack techniques. AI can also help in correlating seemingly unrelated data points to uncover hidden threats.
AI-driven Automated Threat Hunting and Response
Threat hunting involves actively searching for signs of potential threats within an organization’s network and systems. AI-powered threat hunting tools can continuously monitor network traffic, user behavior, and system logs to identify anomalies and suspicious activities.
AI algorithms can automatically identify and prioritize potential threats, allowing security teams to focus their efforts on the most critical issues. Furthermore, AI-driven automation can aid in responding to threats in real-time. For example, if an AI system detects unusual network traffic that matches the behavior of a known malware, it can trigger an automated response to isolate the affected system and prevent further spread.
Additionally, AI-driven threat hunting and response can learn from each incident, improving its accuracy over time and reducing false positives.
By leveraging AI for cyber threat intelligence, organizations can stay ahead of emerging threats, identify vulnerabilities, and respond proactively to potential attacks. This technology enables security teams to work more efficiently, protect sensitive data, and mitigate risks effectively.
AI and Security Operation Centers (SOCs)
Security Operation Centers (SOCs) play a crucial role in monitoring and responding to security incidents within an organization’s IT infrastructure. With the increasing complexity and frequency of cyberattacks, AI has become a game-changer in enhancing the capabilities of SOCs to detect, respond to, and mitigate security threats.
AI-driven Incident Detection and Response
Traditional methods of monitoring security events involve manual analysis of logs and alerts, which can be overwhelming due to the sheer volume of data generated by modern IT environments. AI-driven SOCs leverage machine learning algorithms to analyze vast amounts of data in real-time and identify anomalies or patterns indicative of malicious activities.
AI can detect subtle deviations from normal behavior, helping SOC teams identify unknown threats, zero-day vulnerabilities, and advanced persistent threats that might go unnoticed by conventional security tools. This allows security teams to respond quickly and effectively to potential breaches.
AI-enabled Security Automation and Orchestration
AI not only helps in identifying threats but also automates response actions, reducing the time required to contain and mitigate attacks. AI-enabled security automation can handle routine tasks such as quarantining compromised systems, blocking malicious IP addresses, or isolating infected endpoints.
Furthermore, AI-driven orchestration can streamline incident response workflows by coordinating actions across multiple security tools. For instance, if AI detects a phishing attack, it can trigger an automated response that includes isolating affected systems, sending notifications to users, and initiating further investigation.
Enhancing Threat Intelligence
AI can continuously analyze threat intelligence data from various sources, including internal and external threat feeds, to provide real-time insights into emerging threats. This enables SOCs to proactively identify potential risks and vulnerabilities.
Reducing Alert Fatigue
The sheer volume of alerts generated by security tools can overwhelm SOC analysts and lead to alert fatigue, causing them to miss critical threats. AI can prioritize alerts based on their severity, context, and relevance, reducing false positives and allowing analysts to focus on high-priority incidents.
Continuous Learning and Improvement
AI systems in SOCs can learn from past incidents and response actions, improving their accuracy and effectiveness over time. This iterative learning process ensures that the AI-driven SOC becomes more adept at identifying new threats and adapting to evolving attack techniques.
Incorporating AI into Security Operation Centers not only enhances the overall cybersecurity posture but also allows security teams to respond to threats with greater speed and precision. This technology empowers organizations to detect and mitigate cyber threats efficiently, safeguarding critical data and systems from potential breaches.
AI-driven Vulnerability Management
Vulnerability management is a critical component of cybersecurity that focuses on identifying, assessing, and mitigating security vulnerabilities in an organization’s IT infrastructure. With the increasing complexity of systems and the constant emergence of new vulnerabilities, AI has become a valuable tool in enhancing vulnerability management processes.
AI-powered Vulnerability Assessment
Traditional vulnerability scanning tools often generate a large number of false positives or fail to identify hidden vulnerabilities. AI-driven vulnerability assessment utilizes machine learning algorithms to analyze historical vulnerability data, network configurations, and threat intelligence to identify vulnerabilities with a high degree of accuracy.
AI can prioritize vulnerabilities based on their potential impact and exploitability, enabling security teams to focus on addressing the most critical threats first. This helps organizations allocate resources more effectively and reduce their overall risk exposure.
Risk Prioritization
AI can aid in risk assessment by analyzing various factors such as the asset’s value, its criticality to business operations, and its exposure to potential threats. By considering these factors, AI-driven systems can provide a risk score for each vulnerability, allowing security teams to prioritize mitigation efforts accordingly.
AI-driven Patch Management
Keeping software and systems up-to-date with the latest security patches is essential to prevent known vulnerabilities from being exploited. AI-driven patch management systems can analyze vulnerability data and recommend the most appropriate patches for different systems based on their dependencies and compatibility.
This proactive approach helps organizations apply patches more efficiently and reduces the window of opportunity for attackers to exploit vulnerabilities.
Automated Security Updates
AI can automate the process of applying security updates and patches across an organization’s IT infrastructure. This is particularly beneficial for large-scale environments where manual patch management can be time-consuming and error-prone.
Adapting to Emerging Threats
AI systems in vulnerability management can continuously learn from new threat intelligence and adapt to emerging attack techniques. This allows organizations to stay ahead of attackers by quickly identifying and addressing vulnerabilities that might be targeted in the latest attacks.
Customized Remediation Recommendations
AI-driven vulnerability management systems can recommend specific remediation actions for each vulnerability. These recommendations can include not only patching but also configuration changes or compensating controls to mitigate risks.
Incorporating AI into vulnerability management processes enhances the efficiency and accuracy of identifying and mitigating security risks. By leveraging AI’s capabilities, organizations can better prioritize their efforts, reduce the attack surface, and improve overall cybersecurity posture.
AI in Fraud Detection and Prevention
Fraud detection and prevention are critical challenges for organizations, especially in financial sectors, where fraudulent activities can have severe financial and reputational implications. AI has emerged as a powerful tool to enhance fraud detection capabilities and mitigate risks associated with fraudulent activities.
- AI-powered Fraud Analytics: Traditional rule-based fraud detection systems are often limited in their ability to detect complex and evolving fraud patterns. AI-driven fraud analytics leverage machine learning algorithms to analyze large volumes of data, identifying unusual patterns and anomalies that may indicate fraudulent activities. These algorithms can adapt to new fraud patterns, making them more effective in detecting previously unseen threats.
- Real-time Transaction Monitoring: AI-driven systems can monitor financial transactions in real-time, assessing the risk associated with each transaction. By analyzing historical transaction data and user behavior, AI can flag suspicious transactions for further investigation, minimizing the window of opportunity for fraudulent activities.
- Behavioral Analysis: AI can analyze user behavior and establish a baseline of normal activity. When deviations from this baseline occur, AI systems can trigger alerts for potential fraudulent activities. This approach is particularly effective in detecting account takeovers and insider threats.
- Identity and Access Management (IAM): AI-enabled IAM solutions use machine learning algorithms to analyze user behavior and access patterns. By continuously monitoring user activities, AI can identify unauthorized access attempts or unusual behavior that might indicate identity theft or credential misuse.
- Biometric Authentication: AI-driven biometric authentication systems, such as fingerprint or facial recognition, enhance security by verifying users’ identities based on unique biological characteristics. This reduces the risk of unauthorized access or account takeover due to stolen passwords or credentials.
- Automated Risk Scoring: AI can assign risk scores to users or transactions based on various factors, such as location, transaction history, and device used. Higher-risk transactions or users can undergo additional authentication measures or be subjected to closer scrutiny.
- Reducing False Positives: AI-driven fraud detection systems can significantly reduce the number of false positives compared to traditional rule-based systems. By analyzing more data points and identifying subtle patterns, AI can provide a higher level of accuracy in identifying genuine fraudulent activities.
- Adaptive Learning: AI systems continuously learn from new data, improving their accuracy over time. This is crucial in fraud detection, where attackers frequently change tactics to evade detection.
By leveraging AI in fraud detection and prevention, organizations can effectively combat a wide range of fraudulent activities, safeguard financial transactions, and protect customer data and trust. The adaptability and real-time nature of AI make it a valuable asset in the ongoing battle against ever-evolving fraud tactics.
AI and Cybersecurity Challenges
While AI has shown immense potential in enhancing cybersecurity, it also introduces new challenges that organizations need to address to ensure the effectiveness and reliability of their security measures. Some of the key challenges include:
- AI-based Attacks: Just as AI can be used to strengthen cybersecurity, it can also be exploited by malicious actors to launch more sophisticated attacks. Adversaries can use AI to automate and scale attacks, making them harder to detect and mitigate. For instance, AI-powered malware could adapt its behavior to evade traditional security measures.
- Adversarial Machine Learning: Adversarial machine learning involves manipulating AI systems by introducing small, carefully crafted changes to input data. This can cause AI security systems to misclassify benign inputs as malicious, or vice versa. Defending against adversarial attacks requires developing robust AI models that can accurately classify inputs even in the presence of small perturbations.
- Data Poisoning: AI models used for cybersecurity rely on large datasets for training. If an attacker can inject malicious data into the training dataset, it could lead to the model making incorrect or biased predictions. Ensuring the integrity and quality of training data is crucial to prevent such attacks.
- False Positives and Negatives: AI-powered security systems may produce false positives (flagging legitimate activities as malicious) or false negatives (failing to detect actual threats). Achieving the right balance between accuracy and the frequency of false alarms is a challenge.
- Bias in AI Models: AI models can inherit biases present in the training data. Biased AI systems may lead to unfair or discriminatory decisions, affecting user trust and potentially violating ethical standards.
- Lack of Transparency: Complex AI models, such as deep neural networks, can be challenging to interpret. Lack of transparency in AI decision-making can hinder efforts to understand and address security issues effectively.
- Responsible AI Usage: The deployment of AI in cyber security must align with ethical considerations and responsible AI practices. Overreliance on AI without human oversight can lead to unintended consequences and security vulnerabilities.
- Rapidly Evolving Threat Landscape: As AI evolves, attackers are likely to develop more advanced techniques. Organizations need to continuously update their AI models and defenses to keep pace with evolving threats.
Addressing these challenges requires a multi-faceted approach that combines technical expertise, collaboration, and ethical considerations:
- Robust AI Models: Develop AI models that are resilient to adversarial attacks and can generalize well across different scenarios.
- Continuous Learning: Regularly update AI models with new data to adapt to emerging threats and changing attack patterns.
- Interdisciplinary Collaboration: Collaborate across disciplines, including AI researchers, cybersecurity experts, and ethicists, to ensure a holistic approach to security.
- Ethical AI Development: Prioritize ethical considerations throughout the AI development process, ensuring fairness, transparency, and accountability.
- Human Oversight: Maintain human oversight of AI systems to catch false positives/negatives and make context-based decisions.
- Education and Training: Educate employees about the potential risks and benefits of AI in cybersecurity to enhance awareness and foster responsible usage.
By addressing these challenges, organizations can harness the power of AI to strengthen their cybersecurity posture while upholding ethical standards and maintaining user trust.
Case Studies of AI in Cybersecurity
Certainly, here are some real-world case studies that showcase the effectiveness of AI-powered cybersecurity solutions in thwarting cyber threats:
Darktrace’s Autonomous Response in Identifying Insider Threats
Darktrace, an AI cybersecurity company, implemented an autonomous response system for a large financial institution. The AI system detected unusual network behavior and identified an employee’s compromised credentials. The system then automatically quarantined the affected device and prevented data exfiltration. This incident demonstrated how AI can quickly respond to insider threats and limit potential damage.
Cylance’s AI-Driven Endpoint Protection
Cylance, a cybersecurity company, utilizes AI-driven algorithms to detect and prevent malware and cyber threats. In one instance, Cylance’s AI-based solution identified a previously unknown variant of the WannaCry ransomware. The AI model analyzed the malware’s behavior, allowing it to block the threat even before traditional signature-based solutions could detect and respond to it.
Vectra’s AI-Powered Network Detection and Response
Vectra, a network security company, uses AI to detect and respond to cyberattacks within enterprise networks. In a case involving a manufacturing company, Vectra’s AI platform identified suspicious lateral movements within the network, indicating a potential advanced attack. The AI system automatically alerted security teams, allowing them to isolate the compromised systems and prevent further spread.
FireEye’s AI for Email Threat Detection
FireEye implemented AI-powered solutions to enhance email security. In one instance, a healthcare organization was targeted by spear-phishing emails containing malicious attachments. FireEye’s AI algorithms analyzed email patterns, attachment behavior, and sender reputation to flag the emails as malicious, preventing the malware from being executed.
IBM Watson for Cybersecurity
IBM Watson leverages AI to analyze vast amounts of security data and identify potential threats. In a notable example, IBM Watson identified a strain of malware in a customer’s network that had been previously overlooked by traditional security tools. This discovery allowed the customer to swiftly address the threat and prevent a potential data breach.
SentinelOne’s AI-Powered Endpoint Protection
SentinelOne’s AI-driven platform provides real-time protection against malware and other threats. In a case involving a financial services company, the AI detected suspicious behavior in an employee’s laptop. It prevented the execution of a fileless malware attack and initiated an automated response to contain the threat.
These case studies demonstrate the value of AI-powered cybersecurity solutions in rapidly detecting and responding to a wide range of cyber threats. AI’s ability to analyze large datasets, identify patterns, and adapt to evolving attack methods makes it a valuable asset in the ongoing battle against cybercrime.
Future of AI in Cybersecurity
Certainly, the future of AI in cyber security holds exciting possibilities and innovations. Here are some emerging trends and anticipated developments in AI-driven cybersecurity:
- Advanced Threat Detection and Response: AI will continue to evolve in detecting and responding to advanced and sophisticated cyber threats. Machine learning models will become more adept at identifying anomalies, unknown threats, and zero-day vulnerabilities in real-time. This will help organizations mitigate threats before they can cause significant damage.
- Zero Trust Architecture: AI will play a crucial role in implementing zero trust architecture, where network access is not automatically trusted, regardless of the location of the user or device. AI will continuously monitor user behavior, device health, and network activity to identify potential threats, ensuring a higher level of security.
- Adversarial Machine Learning Defense: As attackers use adversarial machine learning techniques to evade detection, AI will be used in developing defensive models that can identify and counter adversarial attacks. This will lead to a cat-and-mouse game between attackers and defenders, driving innovation on both sides.
- AI-Powered Threat Hunting: AI will enable security teams to proactively hunt for threats by analyzing vast amounts of data to identify hidden patterns and indicators of compromise. AI algorithms will help security professionals to prioritize and investigate potential threats more efficiently.
- Automated Incident Response: AI will increasingly automate incident response actions, such as isolating compromised systems, quarantining malware, and initiating remediation steps. This will reduce response time and minimize the impact of cyber incidents.
- Behavioral Biometrics: AI will enhance authentication and identity management by analyzing user behavior patterns. Behavioral biometrics can detect anomalies in user behavior, identifying potential unauthorized access attempts.
- AI-Enhanced Security Analytics: AI will enable more comprehensive and accurate security analytics. It will sift through massive volumes of data to provide actionable insights, helping organizations identify trends and potential vulnerabilities.
- Securing IoT Devices: With the proliferation of Internet of Things (IoT) devices, AI will be crucial in securing these endpoints. AI-driven anomaly detection can identify compromised devices and prevent them from being used as entry points into networks.
- Privacy-Preserving AI: Privacy concerns are growing, and AI models that can perform analysis on encrypted data without revealing sensitive information will become more prevalent.
- AI in Cyber Training and Simulation: AI-powered simulations and training environments will help organizations simulate cyber attacks and responses, allowing security teams to practice and improve their skills in a controlled setting.
- AI Regulations and Standards: As AI-driven cybersecurity becomes more integral, regulations and standards will emerge to ensure ethical and secure AI usage in defense systems.
The role of AI in the future of cyber defense is poised to be transformative. Organizations that embrace AI-driven cybersecurity technologies will be better equipped to adapt to evolving threats and protect their digital assets effectively.
How Pillar Support Can Help!
Absolutely, Pillar Support is committed to enhancing cyber resilience and safeguarding digital assets through the power of AI. Our AI Conversation Salon is a unique platform where you can engage with AI experts and fellow professionals to discuss and learn about the latest developments in cybersecurity, including fraud awareness and prevention. Here’s how Pillar Support can help:
- AI Conversation Salon: Join our AI Conversation Salon, where you can interact with industry experts and peers to gain insights into the latest trends, technologies, and best practices in cybersecurity and fraud prevention. Engaging in meaningful discussions and sharing experiences will help you stay updated and well-prepared to tackle emerging cyber threats.
- Fraud Awareness Training: Participate in our Fraud Awareness Training sessions, specially designed to equip you with the knowledge and skills to recognize, prevent, and respond to various types of fraudulent activities. Our AI-powered training modules provide real-world scenarios and simulations, enhancing your ability to protect your organization from financial and reputational risks.
- AI-Powered Cybersecurity Solutions: Explore our AI-powered cybersecurity solutions that leverage the latest advancements in AI and machine learning. From threat detection and response to behavioral analytics, our solutions are designed to provide proactive defense against evolving cyber threats.
- Customized Consultation: Pillar Support offers personalized consultation to help your organization assess its cybersecurity needs and develop tailored strategies to enhance cyber resilience. Our experts can guide you in implementing AI-driven solutions that align with your business objectives.
- Access to Resources: Gain access to a wealth of resources, including articles, case studies, webinars, and whitepapers, focused on cybersecurity, fraud prevention, and AI-driven solutions. These resources will empower you with the knowledge needed to make informed decisions.
- Networking Opportunities: Connect with a community of like-minded professionals who are passionate about cybersecurity and AI adoption. Collaborate, share insights, and learn from each other’s experiences to collectively strengthen your cyber defense strategies.
At Pillar Support, we believe that by harnessing the power of AI and fostering a collaborative environment, businesses can effectively combat cyber threats and ensure the security of their digital assets. Join us in our mission to create a safer digital landscape through AI-driven solutions and informed decision-making.
Frequently Asked Questions
What Is AI in Cybersecurity?
AI in cybersecurity refers to the application of artificial intelligence techniques, such as machine learning and deep learning, to enhance and automate various aspects of cybersecurity. AI is used to detect, prevent, and respond to cyber threats in real time by analyzing large volumes of data, identifying patterns, and making informed decisions to mitigate risks.
How Is AI Used in Cybersecurity?
AI is used in cybersecurity for a range of purposes, including:
Threat Detection: AI analyzes network traffic, user behavior, and system activities to identify unusual patterns that may indicate cyber threats.
Anomaly Detection: AI models learn what is normal and can flag deviations from normal behavior, which could be indicative of an attack.
Predictive Analysis: AI can predict potential threats based on historical data and current trends.
Automated Response: AI can automate incident response actions, isolating compromised systems or triggering alerts.
Vulnerability Management: AI can identify vulnerabilities and prioritize them for patching based on their severity and potential impact.
User and Entity Behavior Analytics (UEBA): AI analyzes user behavior to detect suspicious activities and insider threats.
Will AI Replace Cybersecurity?
AI is not expected to replace cybersecurity but rather enhance and transform it. While AI can significantly improve threat detection and response, it still requires human oversight and intervention. Human cybersecurity experts play a critical role in understanding the context of threats, making strategic decisions, and adapting to evolving attack techniques. AI complements human expertise by handling repetitive tasks, analyzing vast amounts of data, and providing rapid response to threats.
AI-driven cybersecurity systems work in tandem with skilled cybersecurity professionals, creating a more robust defense against cyber threats. The human element remains essential for making strategic decisions, understanding the implications of threats, and ensuring ethical considerations are met.
AI is a powerful tool that enhances cybersecurity, but the collaboration between AI systems and human experts remains crucial to effectively protect against cyber threats.